Client
Recruiters are Teach For America’s lifeblood. They spend their time on college campuses across the country, working with juniors and seniors to inspire them to dedicate two years of their lives to Teach For America’s mission.
The challenge
Recruiter-student interactions form the basis of a life-long relationship between Teach For America and a student who chooses to join TFA; therefore, recruiters are also responsible for a great deal of initial data entry in the organization’s CRM as well.
The goal was to find novel ways to reduce the time spent doing data work, and free them up to spend more time doing what recruiters liked about their jobs— building relationships.
My role
Researcher, designer, product owner for cognitive technologies. For many of the studies I designed, I was the sole researcher; however, I did delegate and collaborate with others on the Design team.
Approach
- Interviews
- Contextual studies
- Diary studies
- Rapid prototyping and lean testing
The story of AI and face-to-face interactions
I conducted a foundational research study before we even used the technology. I sat with recruiters in the field and interviewed them to understand how they used first-hand and second-hand knowledge. Out of that research, we formulated a series of rapidly testable concepts that we could test with our machine learning tool set. I worked closely with our Tech Lead and engineering staff to identify the relative effort of the tests. Some of the tests we “mocked” in prototypes, to mimic the outputs a user might expect. Other tests required engineering to simultaneously conduct a “proof of concept.” For those, we often shared real machine learning outputs with users, to assess their reactions.
The goal of these individual research initiatives was to know what was possible and how users responded to what was possible in their day-to-day work.
During the recruiting process, recruiters encounter many of the same document types again. For example, one of their data entry tasks was to process prospect’s resumes. However, those resumes served another function to the recruiters: they read them to get to know the prospect they were going to talk to. In order to alleviate the data entry task, we conducted a pilot wherein we read a student’s resume and entered the data into the CRM.
However, without the resume in front of them— a recruiter needed to be confident in the data. From our initial research we knew that a major “low point” or “trust breaking moment” would be having inaccurate information (e.g. not knowing the correct school the prospect went to) while having coffee with a prospect. Many recruiters would re-check the resume right before talking with a prospect to make sure they didn’t forget anything.
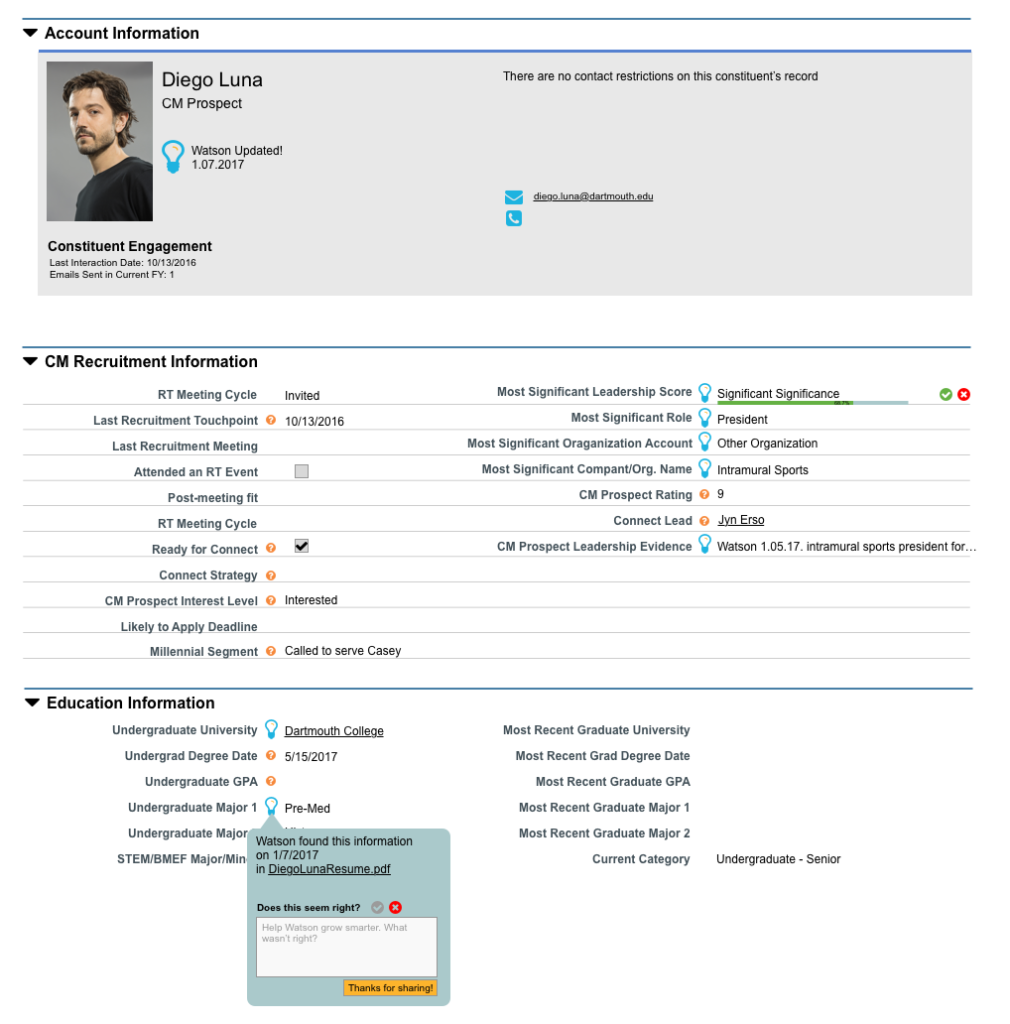
Throughout the three month pilot, we split the teams into two groups. Some recruiters would leverage these new technologies in their day-to-day work. Others on their teams would continue as they had before.
We uncovered a few interesting findings:
Users were less forgiving of the technology. If an intern made the mistake, they would work to correct it, or edit it themselves. They treated the cognitive tool as a machine and expected machine-like precision.
Later in the pilot we gave the machine learning a tool a name. It engendered some degree of empathy. One user said, “No it wasn’t accurate today, she was having a rough day.”
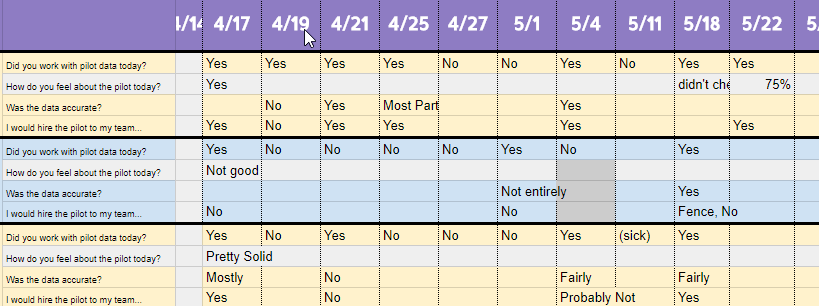
The end results
Despite impressive gains by our engineering team at improving the accuracy of the model, there remained a significant “trust” gap that we could not close. In order to realize time savings, users needed to be sure of the data every time. Because the data was feeding into more than just a CRM— it was feeding into a face-to-face conversation, users were still checking and re-checking the data just as they always had. The risk of a bad interaction was just too great.
We learned a great deal about the potential for efficiency through the use of machine learning; however, this specific user group and context was not the right place to implement this technology further.
P.S. Johanna Warren is a musician that I was listening to a lot while working on this project.